Our lab has a rich history on the development of fast MRI techniques that acquire less k-space data (undersampling) and exploit image properties to reconstruct images without undersampling errors. Previous work was based on compressed sensing to exploit the natural compressibility of medical images to reduce k-space data and accelerate acquisition. Current work is focused on deep learning, where a reconstruction network is learned from multiple examples to map undersampled k-space data to images without undersampling errors. We are developing novel reconstruction network architectures that can highly accelerate the acquisition of 3D MRI – see Figure 1 for an example of 8-fold acceleration of brain MPRAGE to reduce the scan time to only 1 minute. We are also developing reconstruction networks for dynamic motion-resolved 4D MRI which not only reduce scan time but significantly reduce the reconstruction time and enable 4D MRI in a clinical setting – see Figure 2 for an example of movienet which replaces k-space consistency for motion consistency to reduce the reconstruction time to less than 1 second (compressed sensing 4D MRI reconstruction time was higher than 10 minutes).
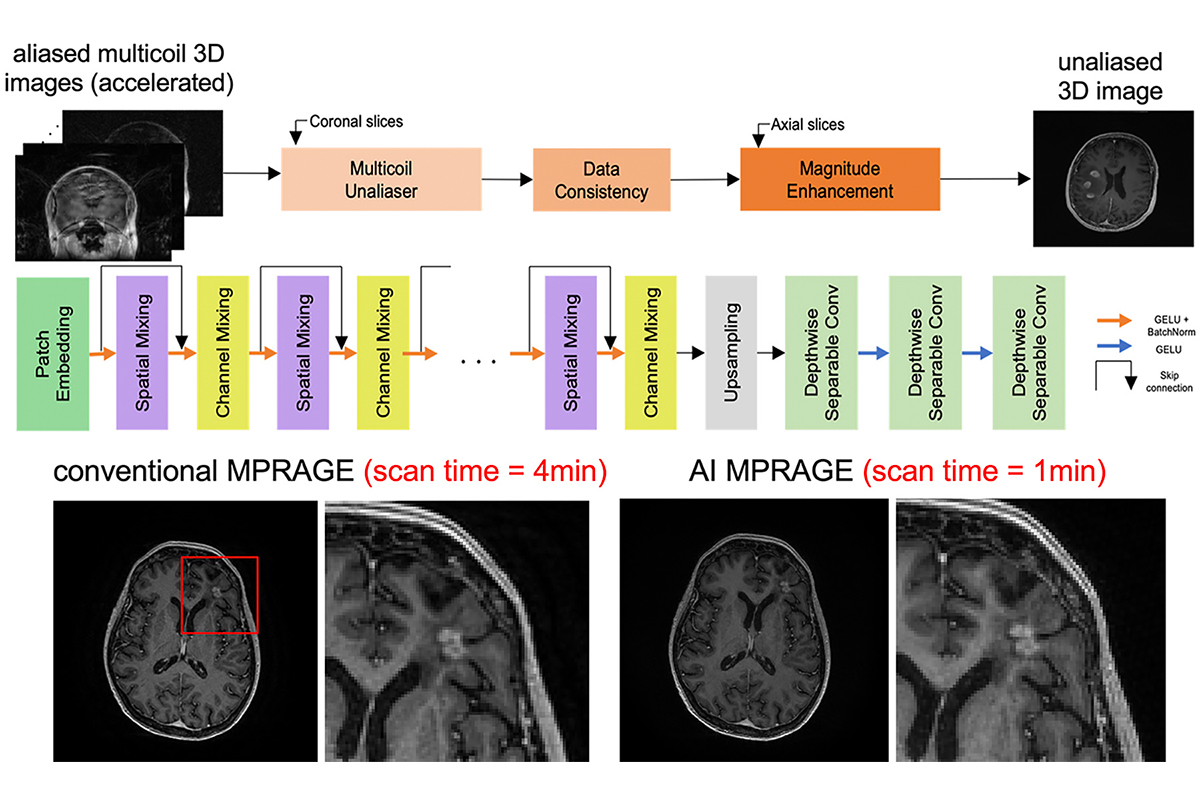
Figure 1: Modular reconstruction network for highly-accelerated 3D MRI based on patch embedding and spatial mixing. The network enables to accelerate brain MPRAGE by factor of 8 and reduce scan time to 1 minute with similar information to conventional MPRAGE with 4-minute scan time.
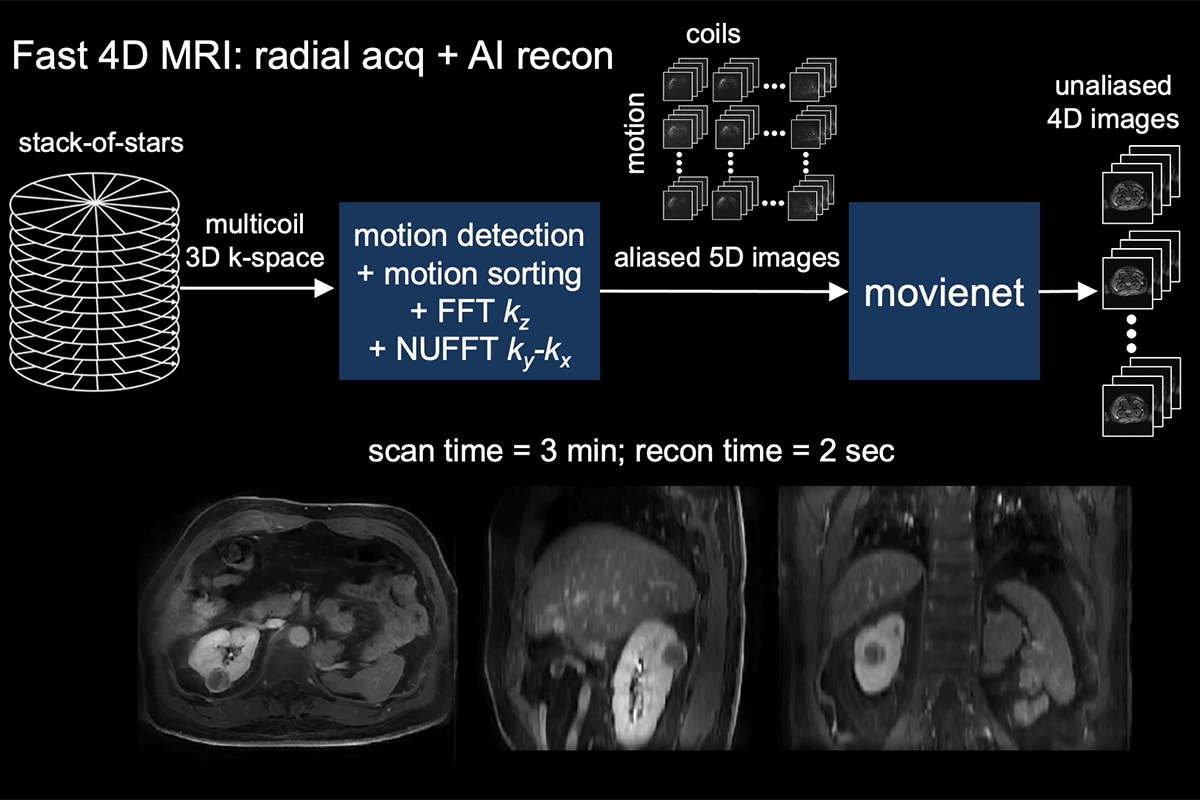
Figure 2: Fast 4D MRI using auto-navigated radial acquisition and AI reconstruction (movienet). In addition to reducing scan time compared to compressed sensing, movienet enabled reconstruction times of only 2 seconds